Multisystem Imaging Recommendations/Guidelines: In the Pursuit of Precision Oncology
CC BY 4.0 · Indian J Med Paediatr Oncol 2023; 44(01): 002-025
DOI: DOI: 10.1055/s-0043-1761266
Abstract
With an increasing rate of cancers in almost all age groups and advanced screening techniques leading to an early diagnosis and longer longevity of patients with cancers, it is of utmost importance that radiologists assigned with cancer imaging should be prepared to deal with specific expected and unexpected circumstances that may arise during the lifetime of these patients. Tailored integration of preventive and curative interventions with current health plans and global escalation of efforts for timely diagnosis of cancers will pave the path for a cancer-free world. The commonly encountered circumstances in the current era, complicating cancer imaging, include coronavirus disease 2019 infection, pregnancy and lactation, immunocompromised states, bone marrow transplant, and screening of cancers in the relevant population. In this article, we discuss the imaging recommendations pertaining to cancer screening and diagnosis in the aforementioned clinical circumstances.
Keywords
AI - BMT - CI-RADS - COIN - COVID-19 - Immunocompromised - iTNM - oncology - Lactation - pregnancyNote
The article is not under consideration for publication elsewhere. Each author participated sufficiently for the work to be submitted. Publication is approved by all authors.
Supplementary MaterialPublication History
Article published online:
06 March 2023
© 2023. The Author(s). This is an open access article published by Thieme under the terms of the Creative Commons Attribution License, permitting unrestricted use, distribution, and reproduction so long as the original work is properly cited. (https://creativecommons.org/licenses/by/4.0/)
Thieme Medical and Scientific Publishers Pvt. Ltd.
A-12, 2nd Floor, Sector 2, Noida-201301 UP, India
Abstract
With an increasing rate of cancers in almost all age groups and advanced screening techniques leading to an early diagnosis and longer longevity of patients with cancers, it is of utmost importance that radiologists assigned with cancer imaging should be prepared to deal with specific expected and unexpected circumstances that may arise during the lifetime of these patients. Tailored integration of preventive and curative interventions with current health plans and global escalation of efforts for timely diagnosis of cancers will pave the path for a cancer-free world. The commonly encountered circumstances in the current era, complicating cancer imaging, include coronavirus disease 2019 infection, pregnancy and lactation, immunocompromised states, bone marrow transplant, and screening of cancers in the relevant population. In this article, we discuss the imaging recommendations pertaining to cancer screening and diagnosis in the aforementioned clinical circumstances.
Keywords
AI - BMT - CI-RADS - COIN - COVID-19 - Immunocompromised - iTNM - oncology - Lactation - pregnancyMultisystem Imaging Recommendations/Guidelines: In the Pursuit of Precision Oncology
Imaging tumor, node, metastasis (iTNM), Cancer Imaging Reporting and Data Systems, Comprehensive Onco-Imaging Network.
Imaging recommendations in special circumstances in oncology—coronavirus disease 2019, pregnancy and lactation, immunocompromised state, screening for cancers, and bone marrow transplant.
Imaging recommendations for artificial intelligence in oncological imaging.
Imaging Tumor, Node, Metastasis, Cancer Imaging Reporting and Data Systems, and Comprehensive Onco-Imaging Network
Addressing the Need
Cancer is a leading cause of morbidity and mortality worldwide, irrespective of the level of human development. As per the estimations of Global Cancer Observatory 2020, approximately 19.3 million new cancer cases and 10 million cancer-related deaths occurred worldwide in 2020.[1] The health care industry is overwhelmed by the sheer number of residual cancer cases and is under immense pressure for not only promptly diagnosing and treating cancer but also developing newer modalities to address the growing needs. Tailored integration of preventive and curative interventions with current health plans and global escalation of efforts for timely diagnosis of cancers will pave the path for a cancer-free world.
With the development of advanced radiological techniques, medical practice is becoming increasingly dependent on imaging. From providing morphological, physiological, to functional information, imaging has grown by leaps and bounds in the past few decades and continues to innovate. Medical imaging plays a significant role in cancer management and directing targeted therapy with a positive influence on the quality-adjusted survival of cancer patients.[2] A simulation-based analysis by Ward et al studied the positive impact of scaling up imaging and treatment availability on the synergistic survival gains for patients with cancer.[3]
The radiology report serves as a document for means of communication between a radiologist and the treating physician or surgeon, describing imaging characteristics of the tumor and providing information on the stage of cancer. For centuries, elaborated and descriptive reporting was the norm in oncoimaging as it allowed the radiologist freedom of expression to emphasize on key findings with the use of free text. However, various pitfalls were identified with narrative reporting. Variability in the length, ambiguity in terminology, and inconsistency in form of the report served as potential sources of confusion among treating oncologists.
Inception of comprehensive synoptic reporting systems has opened up new avenues for a more uniform and simplified approach to oncoimaging. An organized workflow algorithm using structured templates can establish consistency in reports, prevent errors, and promise quality assurance. The use of different categories and subcategories in a report, usually related to organ systems or anatomic structures, can allow clear communication, improve readability, and reduce omission of pertinent information, all of which are expected to contribute to evidence-based medicine.[4]
Since it is well known that imaging can influence the management of cancer by altering the locoregional staging (for example, upstaging of oral cancer by the depiction of mandibular erosion and perineural spread or high infratemporal extension on imaging, both of which are not evident clinically), the introduction of a concise reporting format in oncoimaging is the need of the hour and can be achieved by implementing iTNM staging, i.e., imaging tumor (T), node (N), metastasis (M) staging. Some studies have found that the clinical TNM (cTNM) and the pathological TNM (pTNM) do not always corroborate,[5] [6] highlighting the role of imaging in accurate TNM staging, pre- or posttreatment. A comparative study by Frommhold et al investigated the agreement between pTNM and iTNM in renal tumors; in about 67- cases, iTNM and pTNM were matching, whereas in only 53%-cases, the cTNM matched with pTNM, proving the higher efficacy of imaging in TNM staging.[7] The major drawback of interobserver and intraobserver variations in radiology reporting can be mitigated by standardization.
Reporting and Data Systems
Reporting and Data Systems (RADS) was conceptualized and endorsed by the American College of Radiology (ACR) for providing standardized terminologies and well-defined classification algorithms for concise interpretation of lesions. It is modality and technique dependent and ensures uniformity in lesion description.[8] It uses a stepwise numerical scoring system, based on the degree of suspicion of disease, with management recommendations based on the score. Committees worked to build structured terminology and algorithms to measure the risk of malignancy or disease. The risk assessment criteria are provided in terms such as “normal” or “negative,” “benign,” “probably benign,” “intermediate risk,” to “definitely malignant,” or “high risk.” Tools are provided through a range of products from lexicon, risk stratification system, atlas, flash cards, report templates, and white papers. Certain systems also allow modifiers to convey specific details, such as inadequate examination, negative examination, posttreatment findings, and nondisease-related findings. The prototype system first published by ACR in 1993 was the Breast Imaging Reporting and Data System (BI-RADS) for the stratification of breast cancer patients.[9] Following this, several RADS, oncology, and nononcology have been developed as depicted in [Table 1], and few are under active development with the primary focus on oncological disease.
RADS Disease |
Modality |
|
---|---|---|
BI-RADS |
Breast cancer |
Mammography, MRI, Ultrasound |
C-RADS |
Colon cancer |
CT colonography |
LI-RADS |
Liver cancer |
MRI, CT, US, and contrast-enhanced US |
Lung-RADS |
Lung cancer |
Low dose CT |
NI-RADS |
Head and neck cancers |
PET, CT, MRI |
O-RADS |
Adnexal masses |
Ultrasound |
PI-RADS |
Prostate cancer |
MRI |
TI-RADS |
Thyroid cancer |
Ultrasound |
BT-RADS |
Brain tumor |
MRI |
CAD-RADS |
Coronary artery disease |
CT coronary angiography |
CO-RADS |
COVID |
CT chest |
WHO criteria |
RECIST v1.0 |
RECIST v1.1 |
|
---|---|---|---|
Sum of products of two longest diameters in perpendicular dimensions (bidimensional; surface area) |
Sum of longest diameters of target lesions (unidimensional) |
Sum of longest diameters of nonnodal target lesions and short axis of nodal target lesions (unidimensional) |
|
No. of lesions measured |
All lesions |
Target lesions: maximum 5 per organ, 10 in total |
Target lesions: Maximum 2 per organ, 5 in total |
Nontarget lesions: Not specifically addressed. Increase in size of one or a few nontarget lesions is PD, even when target lesions are stable or responding |
Nontarget lesions: Imaging of nontarget lesions not necessary at every protocol-specified time point for declaration of partial response or stable disease. Increase in nontarget lesions is only PD, if the increase is representative of change in overall tumor burden. |
||
Response |
|||
Complete response (CR) |
No lesion for at least 4 wk |
No lesion for at least 4 wk |
Disappearance of all target lesions or lymph nodes <10> |
Response |
irRC |
irRECIST |
iRECIST |
imRECIST |
---|---|---|---|---|
CR |
Complete disappearance + confirmation not confirmation at mandatory 4 wk |
Confirmation only in nonrandomized trials |
Disappearance of all lesions |
|
PR |
≥30%-decrease + ≥50%-decrease + No unequivocal confirmation at progression in 4 wk nonmeasurable disease |
≥30%-decrease + No unequivocal progression in nonmeasurable disease |
≥30%-decrease |
|
PD |
>20%-increase + ≥25%-increase + > 5 mm absolute confirmation at increase in MTB 4 wk + confirmation at 4 wk |
Immune unconfirmed progressive disease (iUPD) and immune confirmed progressive disease (iCPD) |
≥20%-increase or > 5 mm absolute increase |
|
SD |
None |
None |
None |
None |
Response assessment criteria |
Year |
Imaging modalities |
Assessment type |
Advantages |
Disadvantages |
---|---|---|---|---|---|
WHO |
1979 and 1981 |
CT |
Anatomic, size-based |
First objective measurements of images of all lesions |
Time- consuming procedure; interobserver variability |
RECIST v1.0 |
2000 |
CT, MRI |
Anatomic, size-based |
Easier than WHO; measurement of “target” and “nontarget” lesions; less measurement errors |
Only anatomic assessment |
RECIST v1.1 |
2009 |
CT, MRI, PET |
Anatomic, size-based |
Easier than RECIST v1.0 Lymph nodes incorporated |
Only anatomic assessment |
mRECIST |
2006 |
CT, MRI |
Anatomic, size-based |
Simpler than RECIST v1.1 |
Only anatomic assessment, not prospectively validated |
mRECIST for HCC |
2010 |
CT, MRI |
Anatomic and functional; based on contrast enhancement |
Measurement of a viable tumor. Appropriate for loco-regional therapies |
Only for HCC |
EASL and qEASL |
2000 and 2012 |
CT, MRI |
Anatomic and functional; based on contrast enhancement |
qEASL is better than RECIST to predict OS; measurement of a viable tumor |
Only for HCC |
Choi criteria |
2007 |
CT |
Anatomic and functional; based on tumor density |
Validated for GIST, more precise than RECIST; Measurement of a viable tumor |
Only for GIST |
Morphologic response |
2009 |
CT |
Anatomic and functional; based on morphologic changes |
Appropriate for bevacizumab treatment |
For CRC liver metastases, not prospectively validated |
irRC |
2009 |
CT, MRI |
Anatomic, size-based |
For the treatment with immune- checkpoint inhibitors, capture of atypical response (pseudoprogression) |
The variability of interpretation |
irRECIST |
2013 |
||||
iRECIST |
2017 |
||||
imRECIST |
2018 |
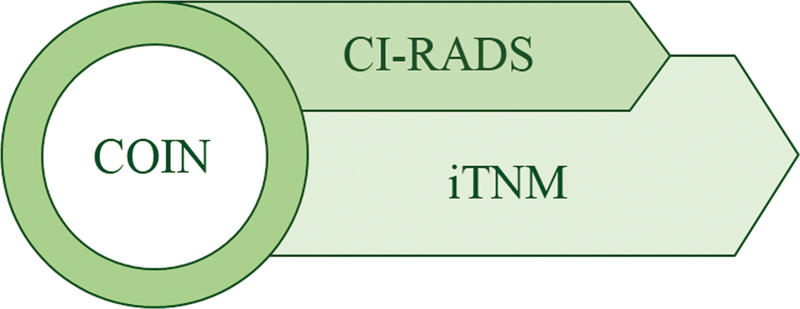
| Figure 1:
Cancer Imaging Recommendations in Special Circumstances—Coronavirus Disease 2019, Pregnancy and Lactation, Immunocompromised State, Screening for Cancers, and Bone Marrow Transplant
Coronavirus Disease and Cancer
Globally, by the end of May 2022, there have been 525,467,084 confirmed cases of COVID- 19, including 6,285,171 deaths, reported to WHO. Patients diagnosed with, suspected of, or at risk of developing cancer are especially vulnerable during this pandemic as there can be delay in early detection, delay in treatment initiation, and progression of cancer[35] [36] These patients have more adverse outcomes as compared to the general population due to COVID-19 induced immunosuppression.[37]
Coronavirus Disease Imaging
Cancer treatments like chemotherapy and immunosuppressant taken after surgical cancer removal usually weaken the patient's immune system rendering them more vulnerable COVID infection.[38] Among cancer patients, patients with hematolymphoid malignancy have a maximum risk of getting affected by COVID.[39] Lung ultrasound and CT have a high sensitivity in detecting pulmonary interstitial involvement.[40] Chest radiography is an easily available and affordable tool in COVID care but it is less sensitive for early lung changes due to infection.[41] [Table 5] summarizes the indication and common findings of various imaging modalities.
Imaging Indication Common findings modality |
||
---|---|---|
Lung ultrasound (LUS) |
Triage Severity of lung damage Evolution of the disease Safely used in children and pregnant women |
B-Line Pleural line irregularity White lung Consolidation Broncho-grams |
CT |
More sensitive and specific |
GGO GGO + Consolidation Crazy paving Broncho-grams Reversed halo sign |
Chest X-ray |
Less sensitive than a CT scan, it may be used as a first-line approach In very critical patients |
Bilateral consolidation GGO White out lungs |
MRI |
Not relevant for the evaluation of lung disease |
Diagnostic pathway COVID-induced thrombogenic acute stroke, impaired consciousness, acute necrotizing hemorrhagic encephalopathy |
FDG-PET |
Not used in an emergency |
Cancer staging |
Impact on imaging |
Recommendation |
|
---|---|---|
Lung imaging |
COVID-19 lung findings can mimic therapy- associated pneumonitis and other viral infections. 18F-FDG uptake in mediastinal lymph nodes in a patient with COVID-19 has been described, consistent with active inflammation |
Discussion with treating clinician, careful history, and appropriate evaluation for infection should be considered. |
Neurologic imaging |
Ischemic and hemorrhagic complications due hypercoagulopahthy. Meningoencephalitis, demyelinating lesions and acute leukoencephalopathy. Can rarely confused with immunotherapy-associated or tumor induced autoimmune and/or limbic encephalitis. |
Assessing the exact etiology of brain imaging findings inpatients on immunotherapy and COVID-19 is suggested. |
Abdominal findings |
Abdominal manifestations in patients result in imaging findings most of which are nonspecific. |
No evidence suggesting mimic of cancer. |
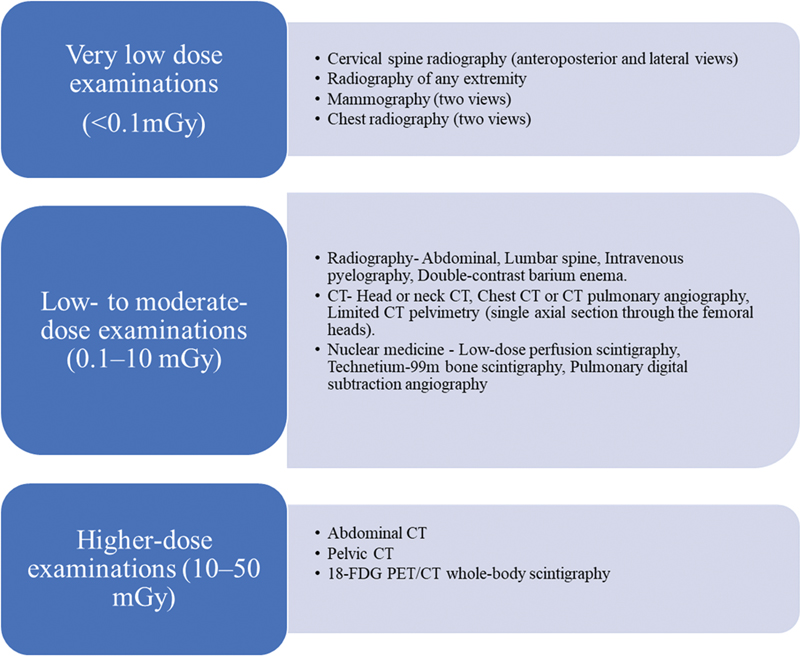
| Figure 1:Radiation doses associated with common radiologic examinations.
Recommendations
The following recommendations are made regarding diagnostic imaging methods during pregnancy and breastfeeding by the Committee on Obstetric Practice of the American College of Obstetricians and Gynecologists[63]:
The preferred imaging methods for pregnant patients are ultrasound and MRI, which are both low risk. However, these methods should only be utilized carefully and when they are anticipated to provide the patient with medical benefits.
With very few instances, radiation exposure by radiography, CT scans, or nuclear medicine imaging methods is at a dose significantly lower than the exposure linked to harm to fetuses. A pregnant patient should not be denied access to these procedures if they are required in addition to ultrasonography or MRI or are more accessible for the diagnostic at hand.
Gadolinium contrast should only be used sparingly in MRI procedures; it should not be utilized as a contrast agent in pregnant women unless it greatly enhances diagnostic accuracy and is anticipated to have positive effects on the fetus or the mother.
Gadolinium administration should not be followed by a break in breastfeeding.
Imaging Recommendations for Bone Marrow Transplant
Bone marrow transplantation (BMT)/hematopoietic stem cell transplantation is the procedure in which patient's diseased stem cells or stem cells destroyed due to the high dose of chemotherapy/radiotherapy are replaced by healthy stem cells. BMT destroys tumor cells in case of malignancy and replaces dysfunctional cells by generating functional cells in nonmalignant hematological disorders (immune deficiency syndromes and hemoglobinopathies).
Indications
Broadly there are three indications of BMT: (1) curative for certain types of hematological malignancies, (2) supportive for those undergoing high-dose chemotherapy, and (3) nonmalignant hematological disorders.[64] [65] The various indications are enumerated in [Table 7].[64] [66]
Various Indications for BMT |
---|
1. Acute lymphoblastic leukemia (ALL) |
2. Acute myeloid leukemia (AML) |
3. B-cell lymphomas |
4. Chronic lymphocytic leukemia (CLL)/small lymphocytic lymphoma |
5. Chronic myeloid leukemia (CML) |
6. Gestational trophoblastic neoplasia (GTN) |
7. Hodgkin lymphoma (HL) 8. Multiple myeloma (MM) |
9. Myelodysplastic syndromes (MDS) |
10. Myeloproliferative neoplasms |
11. Primary cutaneous lymphoma |
12. T-cell lymphomas |
13. Germ cell tumors (testicular tumors) refractory to chemotherapy |
14. Systemic light chain amyloidosis |
15. Systemic mastocytosis |
16. Waldenstrom macroglobulinemia |
17. Non-malignant hematological disorders, e.g., severe combined immune deficiency syndrome (SCID), thalassemia, sickle cell anemia |
18. Other diseases: Chronic granulomatous disease, leukocyte adhesion deficiency, Chediak–Higashi syndrome, Kostman syndrome, Fanconi anemia, Blackfan–Diamond anemia, and enzymatic disorders. |
Organs affected |
Complications |
---|---|
Pulmonary complications |
Preengraftment phase -Fungal infection -Diffuse alveolar hemorrhage -Pulmonary edema -Engraftment syndrome Early posttransplantation phase -Cytomegalovirus infection -Pneumocystis jiroveci pneumonia -Idiopathic pneumonia syndrome Late posttransplantation phase -Bronchiolitis obliterans -Cryptogenic organizing pneumonia |
Hepatic complications |
-Acute GVHD -Drug-induced hepatotoxicity -Viral hepatitis -Liver abscess -Hepatic sinusoidal obstruction syndrome |
Gastrointestinal complications |
-GVHD (acute and chronic) -Neutropenic enterocolitis |
Genitourinary complications |
-Renal function impairment -Hemorrhagic cystitis -Renal parenchymal infections |
Central nervous |
-CNS infections |
system (CNS) |
-Intraaxial hematomas |
complications |
-Infarction -Posterior reversible encephalopathy syndrome |
Musculoskeletal complications |
-Osteoporosis -Avascular necrosis |
Secondary malignancies |
-Solid tumors -Hematological malignancies -Posttransplant lymphoproliferative disease |
Imaging studies |
Indications |
---|---|
Radiography |
• In suspected Engraftment Syndrome and pulmonary edema. • Postline placement to identify the position and to evaluate any complications such as a pneumothorax. |
CT Chest |
• In suspected lung infection, diffuse alveolar hemorrhage and idiopathic pneumonia syndrome. • In suspected chronic GVHD, posttransplant lymphoproliferative disorder (PTLD) and veno-occlusive disease (late posttransplant period) • I.V. contrast study is recommended for imaging in venoocculusive disease. |
USG abdomen |
• In suspected sinusoidal obstruction syndrome, Budd–Chiari syndrome, neutropenic colitis, pyelonephritis, and hemorrhagic cystitis. |
CT abdomen with contrast |
• In suspected acute GVHD and infection. • In suspected PTLD and chronic GVHD (late posttransplant period). |
CT brain |
• In suspected intracranial hemorrhages, PRES, and infection. • I.V Contrast study is recommended for imaging in infection. |
MRI Brain |
• In suspected metabolic encephalopathy, PRES and infection. • Post-HSCT carcinogenesis (late posttransplant period) • I.V. contrast study is recommended for imaging in infection and post-HSCT carcinogenesis. |
Age group and risk assessment |
Recommendations |
---|---|
40–44 y |
Choice to start annual breast screening should be given explaining the risks and potential benefits. |
45–49 y |
Annual mammograms |
50–54 y |
Clinical breast examination with annual mammograms |
55–74 y |
Clinical breast examination with mammograms every 2 y, choice to continue yearly screening. |
75 y and older |
Screening should continue as long as a woman is in good health and is expected to live 10 more years or longer. |
Women at higher-than-average risk (family history or with predisposing genetic mutation) |
MRI and mammogram every year |
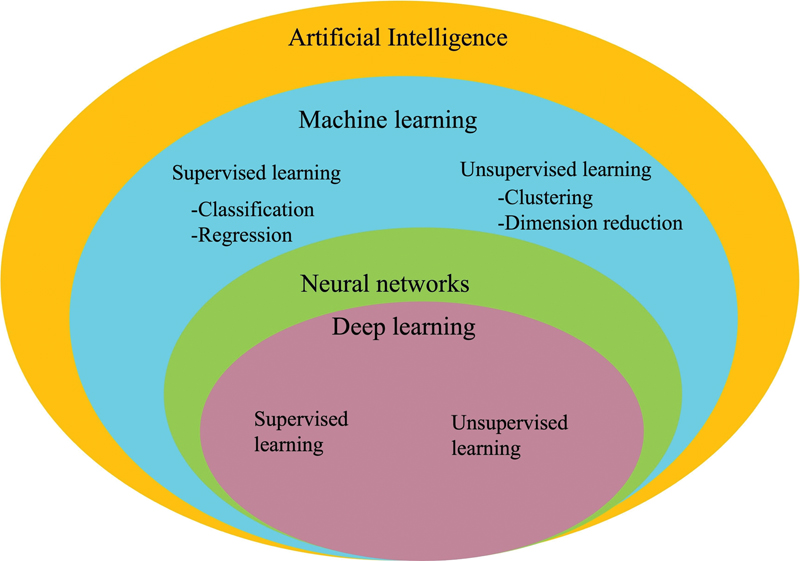
| Figure 2:Relationship between artificial intelligence, machine learning (ML), neural network, and deep learning (DL).
Radiomics combined with the ML model |
DL |
---|---|
Large data required, but can work in lesser data in comparison to DL. |
Cannot perform without huge data. |
Can work on low-end machines |
Need high-end machines to process high data. |
For solving simple and less complex problems. |
For solving complex problems. |
Model training takes less time but validation requires a longer time |
Model training requires a longer time but validation is less time consuming. |
Radiomics uses manual feature extraction step to proceed further. |
DL autolearns from data, so manual feature extraction step not required. |
Result interpretation and reasoning is comprehensible. |
As DL autolearns and has many hidden layers, reasoning behind result is not comprehensible. |
Applications of Artificial Intelligence in Oncology
Screening, diagnosis, lesion characterization (e.g., classification task into benign or malignant), prediction of tumor genome status, response to therapy, prognosis, outcome and recurrence prediction are the major applications of AI (radiomics, ML, and DL) in oncology on imaging.[112] [113] [114] [115] Besides, pretrained DL models can be used to perform automatic segmentation (delineation of tumor boundaries). [Fig. 3] depicts the overview of AI application in oncological imaging.

| Figure 3:Applications of artificial intelligence in oncological imaging.
Few studies involving AI in cancer diagnosis and management include:
Histology prediction and screening of breast cancer on mammography.[122] [123]
Lung nodule segmentation on computed tomography (CT).[128] [129] [130]
Prostate gland tumor detection on magnetic resonance imaging (MRI).[112] [132] [133]
Glioblastoma recurrence prediction.[137]
DL Workflow in Radiology
A DL-based study should be undertaken if it has the potential to alter patient management, and sufficient data are available for its execution. A typical DL workflow comprises of the following steps.
Collaboration: Radiologist, clinician, software developers (technical expertise), and data scientist need to collaborate for the execution of a DL-based study.[115]
Ethics committee approval: Approval of institutional ethics committee should be sought.[115]
Image acquisition and data deidentification: CT, PET, MRI, ultrasonography (USG), radiographs, and mammogram can be used for image acquisition based on the requirement of the study. Images should be anonymized to remove patient identity and should be exported as Digital Imaging and Communication in Medicine file.[115] Alternatively, imaging biobanks, which are open source image data repository, can be used for research.[138] Images should be annotated for training the DL models.
Nonimaging data collection and curation: This includes other nonimaging data that need to be collected, for example, clinical data, radiology, and pathology reports.
Segmentation: Automatic and semiautomatic segmentation, that is, DL-based delineation of tumor boundaries, can be achieved, after training models for segmentation.[115] [117] [139]
Model training, validation, and testing: DL autoextracts features from imaging data. Appropriate model should be selected after training based on performance using the receiver operating characteristic (ROC) curve and area under the ROC curve. The model should be fine-tuned on validation datasets. Test dataset should be used for evaluating the performance of a model for practical deployment.[115]
Hardware selection: It is based on the quantity of data available and the complexity of model. Central processing unit has huge memory but limited bandwidth, whereas graphics processing unit (GPU) and tensor processing units (TPU) have limited memory but high bandwidth.[115]
Radiomics Workflow
For a radiomic study, as a general rule, 10 to 15 samples per feature are required for classification studies, though the number of features cannot be predetermined.[113] After institutional ethics committee approval, the following steps should be followed for a radiomic study.
Image acquisition and data curation: It is the same as described in DL workflow.
Segmentation and feature stability: Region of interest (ROI) is drawn within the tumor, or peritumoral zones, in two dimension (2D) or 3D. With manual segmentation, radiomic features sensitive to interreader variations should be rejected. The intraclass correlation coefficient should be used to reject nonreproducible features after repeating tumor segmentation by one or more readers.[113] [140]
Image preprocessing: Raw image data need to be homogenized and enhanced before radiomic features can be extracted.[3] [30] Various preprocessing steps include signal intensity (SI) normalization, image interpolation, range resegmentation, denoising, bias field correction, motion correction, image thresholding, and discretization.[113] [140]
Feature extraction: It refers to calculation of features using feature descriptors to quantify characteristics of gray levels within ROI in accordance with Image Biomarker Standardization Initiative guidelines.[140] In radiomics, feature extraction is handcrafted that is chosen by a data scientist.[116] The various feature classes are as follows.
I. Morphologic features: It includes volume, diameter, area, and elongation features.
II. Intensity-based features (first order features): This describes distribution of intensities within an ROI and are further grouped based on location, spread, and shape of distribution. Images from MRI and USG require standardization before calculation of first order features as they generate arbitrary intensity images.
III. Texture features (second order features): In this, spatial location as well as signal intensities are used for calculating features.
IV. Higher order features: These are imaging features acquired after applying filters or mathematical transforms using statistical methods.[141]
[Table 12] describes the various feature extraction classes.[113] [141]
Feature class |
Description |
---|---|
Morphologic features |
Volume, diameter, area, sphericity can be quantitative or descriptive. |
Intensity-based features (first-order features) |
These features measure a. location of distribution (mean, median, mode). b. Spread of distribution (variance, interquartile range). c. Shape of distribution (skewness, kurtosis). |
Texture features (second order features): |
These describe spatial complexity and relationships of SI between adjacent pixels. These include gray-level co-occurrence matrix, gray-level run-length matrix, gray-level size-zone matrix, gray-level distance-zone matrix, neighborhood gray-tone difference matrix, and |
Systematic Review and Meta-Analysis Data
A systematic review of 734 original studies on applied ML in patient diagnosis, classification, and prognostication studies from January 2016 to December 2020 concluded that ML has helped in understanding the principles underlying oncogenesis and in serving as a noninvasive biomarker for cancer diagnosis, prognosis, prevention, and treatment; however, robustness and explainability of the models need to be improved.[152] Another systematic review from articles published between 2009 and April 2021 on AI techniques in cancer diagnosis and prediction revealed 13 articles on breast cancer, 10 articles on brain tumors, 8 articles each on cervical, liver, lung, and skin cancers, 7 articles on stomach cancer, 6 articles on colorectal cancer, 5 articles each on renal and thyroid cancers, 2 articles each on oral and prostate cancers, and 1 article each on neuroendocrine tumors and lymph node metastasis.[153]
Summary of Recommendations
AI-based research in imaging is a collaborative effort of radiologists, clinician, software developers (technical expertise), and data scientist, and it should be undertaken only if it has the potential to alter patient management as it involves additional workforce and consumes a lot of time.
Anonymization of patient images and clinical data is a compulsory step of AI-based research.
Open-source data (imaging biobanks) should be encouraged after proper deidentification, to cater to the need of huge data requirements and so as to benefit a larger population worldwide. As little medical data should be retained as reasonably acceptable. Transfer learning may be employed when there is data constraint.
Developed AI model should be appropriately validated prior to its deployment in an institution. Federated learning can help in validation and building a robust model.
Updated data storage systems and data encryption is a necessity to prevent data breach.
Standard operating procedure for AI workflow, and data sharing and ethics, are attached in the [Supplementary Material Figure 1] and [2].
Conflict of Interest
None declared.
Note
The article is not under consideration for publication elsewhere. Each author participated sufficiently for the work to be submitted. Publication is approved by all authors.
Supplementary MaterialReferences
- ung H, Ferlay J, Siegel RL. et al. Global Cancer Statistics 2020: GLOBOCAN estimates of incidence and mortality worldwide for 36 cancers in 185 countries. CA Cancer J Clin 2021; 71 (03) 209-249
- iles K. Can imaging help improve the survival of cancer patients?. Cancer Imaging 2011; 11 Spec No A (1A): S86-S92
- ard ZJ, Scott AM, Hricak H. et al. Estimating the impact of treatment and imaging modalities on 5-year net survival of 11 cancers in 200 countries: a simulation-based analysis. Lancet Oncol 2020; 21 (08) 1077-1088
- immermans S, Mauck A. The promises and pitfalls of evidence-based medicine. Health Aff (Millwood) 2005; 24 (01) 18-28
- deedo A, Van Schil P, Corthouts B, Van Mieghem F, Van Meerbeeck J, Van Marck E. Comparison of imaging TNM [(i)TNM] and pathological TNM [pTNM] in staging of bronchogenic carcinoma. Eur J Cardiothorac Surg 1997; 12 (02) 224-227
- ahajan A, Suthar M, Agarwal U, Shukla S, Thiagarajan S, Sable N. Imaging-based T stage (iT) as a predictive and prognostic marker for outcome in T4 stage tongue carcinomas: a narrative review. Cancer Res Stat Treat 2021; 4 (04) 677-683
- rommhold J, Jocham D, Doehn C. Wie gut ist die Korrelation zwischen klinischen und pathologischen TNM-Stadien bei Nierentumoren?. [How accurate is the correlation between clinical and pathological TNM stages in rena l tumours?] Aktuelle Urol 2011;42(4):247–251. German. doi: 10.1055/s-0031-1271392. Epub 2011 Jun 29
- n JY, Unsdorfer KML, Weinreb JC. BI-RADS, C-RADS, CAD-RADS, LI-RADS, Lung-RADS, NI-RADS, O-RADS, PI-RADS, TI-RADS: Reporting and Data Systems. Radiographics 2019; 39 (05) 1435-1436
- ccessed December 30, 2022 at: https://www.acr.org/Clinical-Resources/Reporting-and-Data-Systems/Bi-Rads
- Mahajan A. Synoptic reporting in lung cancers using Lung Cancer Reporting and Data System (LC-RADS): the road ahead for standardization of imaging in lung cancer staging. Cancer Res Stat Treat 2021; 4 (01) 61-66
- Mahajan A, Agarwal U, Padashetty S. et al. A narrative review of the role of cross-sectional imaging in the management of thyroid carcinoma: imaging guidelines and T-CIRADS. Cancer Research, Statistics, and Treatment 2022; 5 (03) 490-498
- Mahajan A, Agarwal U, Gupta A. et al. Synoptic reporting in head and neck cancers—Head and Neck Cancer Imaging Reporting and Data Systems (HN-CIRADS): the journey ahead for standardization of imaging in head and neck cancer staging. Cancer Res Stat Treat 2022; 5 (02) 322-330
- Ayer T, Chen Q, Burnside ES. Artificial neural networks in mammography interpretation and diagnostic decision making. Comput Math Methods Med 2013; 2013: 832509
- Steyerberg EW. Clinical Prediction Models: A Practical Approach to Development, Validation, and Updating. New York: Springer; 2009
- Carrino JA, Ohno-Machado L. Development of radiology prediction models using feature analysis. Acad Radiol 2005; 12 (04) 415-421
- European Society of Radiology (ESR). ESR position paper on imaging biobanks. Insights Imaging 2015; 6 (04) 403-410
- Vaidya T, Agrawal A, Mahajan S, Thakur MH, Mahajan A. The continuing evolution of molecular functional imaging in clinical oncology: the road to precision medicine and radiogenomics (Part II). Mol Diagn Ther 2019; 23 (01) 27-51 Erratum in: Mol Diagn Ther. 2018 Nov 20; PMID: 30387041
- Miller AB, Hoogstraten B, Staquet M, Winkler A. Reporting results of cancer treatment. Cancer 1981; 47 (01) 207-214
- Therasse P, Arbuck SG, Eisenhauer EA. et al. New guidelines to evaluate the response to treatment in solid tumors. European Organization for Research and Treatment of Cancer, National Cancer Institute of the United States, National Cancer Institute of Canada. J Natl Cancer Inst 2000; 92 (03) 205-216
- Kim JH. Comparison of the EORTC criteria and PERCIST in solid tumors: a pooled analysis and review. Oncotarget 2016; 7 (36) 58105-58110
- Mahajan A, Cook G. Clinical applications of PET/CT in oncology. In: Basic Science of PET Imaging; 2017: 429
- Mahajan A, Cook G. Physiologic and molecular basis of PET in cancer imaging. In: Basic Science of PET Imaging; 2017: 399-427
- Ko CC, Yeh LR, Kuo YT, Chen JH. Imaging biomarkers for evaluating tumor response: RECIST and beyond. Biomark Res 2021; 9 (01) 52
- Somarouthu B, Lee SI, Urban T, Sadow CA, Harris GJ, Kambadakone A. Immune-related tumour response assessment criteria: a comprehensive review. Br J Radiol 2018; 91 (1084): 20170457
- Choi H, Charnsangavej C, Faria SC. et al. Correlation of computed tomography and positron emission tomography in patients with metastatic gastrointestinal stromal tumor treated at a single institution with imatinib mesylate: proposal of new computed tomography response criteria. J Clin Oncol 2007; 25 (13) 1753-1759
- Lencioni R, Llovet JM. Modified RECIST (mRECIST) assessment for hepatocellular carcinoma. Semin Liver Dis 2010; 30 (01) 52-60
- Wahl RL, Jacene H, Kasamon Y, Lodge MA. From RECIST to PERCIST: evolving considerations for PET response criteria in solid tumors. J Nucl Med 2009; 50 (Suppl. 01) 122S-150S
- Chinot OL, Macdonald DR, Abrey LE, Zahlmann G, Kerloëguen Y, Cloughesy TF. Response assessment criteria for glioblastoma: practical adaptation and implementation in clinical trials of antiangiogenic therapy. Curr Neurol Neurosci Rep 2013; 13 (05) 347
- Cheson BD, Pfistner B, Juweid ME. et al; International Harmonization Project on Lymphoma. Revised response criteria for malignant lymphoma. J Clin Oncol 2007; 25 (05) 579-586
- Abdel-Sattar MH, Abdelaziz O, Othman AO, El-Refaei SM. The use of Deauville criteria in follow-up assessment of response to therapy in extra-nodal Non-Hodgkin's lymphoma. Egypt J Radiol Nucl Med 2018; 49 (01) 209-215
- Nielsen N, Nielsen A, Gerke O, Juul-Jensen K, Larsen T, Hildebrandt M. A head-to-head comparison of the Lugano Classification and PERCIST for FDG-PET/CT response assessment in diffuse large B-cell lymphoma. J Nucl Med 2021; 62 (Suppl 1): 1688
- Costelloe CM, Chuang HH, Madewell JE, Ueno NT. Cancer response criteria and bone metastases: RECIST 1.1, MDA and PERCIST. J Cancer 2010; 1: 80-92
- Aykan NF, Özatlı T. Objective response rate assessment in oncology: current situation and future expectations. World J Clin Oncol 2020; 11 (02) 53-73
- Tirkes T, Hollar MA, Tann M, Kohli MD, Akisik F, Sandrasegaran K. Response criteria in oncologic imaging: review of traditional and new criteria. Radiographics 2013; 33 (05) 1323-1341
- Ranganathan P, Sengar M, Chinnaswamy G. et al; National Cancer Grid of India. Impact of COVID-19 on cancer care in India: a cohort study. Lancet Oncol 2021; 22 (07) 970-976
- Richards M, Anderson M, Carter P, Ebert BL, Mossialos E. The impact of the COVID-19 pandemic on cancer care. Nat Can 2020; 1 (06) 565-567
- Johannesen TB, Smeland S, Aaserud S. et al. COVID-19 in cancer patients, risk factors for disease and adverse outcome, a population-based study from Norway. Front Oncol 2021; 11: 652535
- Haghighat S, Dehghani M. COVID-19 in immunosuppressed and cancer patients: a review. Asian Pacific J Cancer Care 2020; 5 (S1): 69-73
- Fillmore NR, La J, Szalat RE. et al. Prevalence and outcome of COVID-19 infection in cancer patients: A National Veterans Affairs Study. J Natl Cancer Inst 2021; 113 (06) 691-698
- Aljondi R, Alghamdi S. Diagnostic value of imaging modalities for COVID-19: scoping review. J Med Internet Res 2020; 22 (08) e19673
- Cleverley J, Piper J, Jones MM. The role of chest radiography in confirming covid-19 pneumonia. BMJ 2020; 370: m2426
- Nnaji CA, Moodley J. Impact of the COVID-19 pandemic on cancer diagnosis, treatment and research in African health systems: a review of current evidence and contextual perspectives. Ecancermedicalscience 2021; 15: 1170
- Kulkarni T, Sharma P, Pande P, Agrawal R, Rane S, Mahajan A. COVID-19: a review of protective measures. Cancer Res, Statistics Treat 2020; 3 (02) 244-253
- Curigliano G, Banerjee S, Cervantes A. et al; Panel Members. Managing cancer patients during the COVID-19 pandemic: an ESMO multidisciplinary expert consensus. Ann Oncol 2020; 31 (10) 1320-1335
- Boettcher AN, Hammoud DA, Weinberg JB, Agarwal P, Mendiratta-Lala M, Luker GD. Cancer imaging and patient care during the COVID-19 pandemic. Radiol Imaging Cancer 2020; 2 (06) e200058
- McCollough CH, Schueler BA, Atwell TD. et al. Radiation exposure and pregnancy: when should we be concerned?. Radiographics 2007; 27 (04) 909-917 , discussion 917–918
- Patel SJ, Reede DL, Katz DS, Subramaniam R, Amorosa JK. Imaging the pregnant patient for nonobstetric conditions: algorithms and radiation dose considerations. Radiographics 2007; 27 (06) 1705-1722
- American Institute of Ultrasound in Medicine. Statement on the safe use of Doppler ultrasound during 11–14 week scans (or earlier in pregnancy). Laurel, MD: AIUM; 2011. Accessed December 30, 2022 at: http://www.aium.org/officialStatements/42
- Chen MM, Coakley FV, Kaimal A, Laros Jr RK. Guidelines for computed tomography and magnetic resonance imaging use during pregnancy and lactation. Obstet Gynecol 2008; 112 (2 Pt 1): 333-340
- Leyendecker JR, Gorengaut V, Brown JJ. MR imaging of maternal diseases of the abdomen and pelvis during pregnancy and the immediate postpartum period. Radiographics 2004; 24 (05) 1301-1316
- Kanal E, Barkovich AJ, Bell C. et al; Expert Panel on MR Safety. ACR guidance document on MR safe practices: 2013. J Magn Reson Imaging 2013; 37 (03) 501-530
- De Santis M, Straface G, Cavaliere AF, Carducci B, Caruso A. Gadolinium periconceptional exposure: pregnancy and neonatal outcome. Acta Obstet Gynecol Scand 2007; 86 (01) 99-101
- Ray JG, Vermeulen MJ, Bharatha A, Montanera WJ, Park AL. Association between MRI exposure during pregnancy and fetal and childhood outcomes. JAMA 2016; 316 (09) 952-961
- Sachs HC. Committee On Drugs. The transfer of drugs and therapeutics into human breast milk: an update on selected topics. Pediatrics 2013; 132 (03) e796-e809
- De Santis M, Cesari E, Nobili E, Straface G, Cavaliere AF, Caruso A. Radiation effects on development. Birth Defects Res C Embryo Today 2007; 81 (03) 177-182
- Brent RL. Saving lives and changing family histories: appropriate counseling of pregnant women and men and women of reproductive age, concerning the risk of diagnostic radiation exposures during and before pregnancy. Am J Obstet Gynecol 2009; 200 (01) 4-24
- Wagner LK, Applegate K. American College of Radiology. ACR practice guideline for imaging pregnant or potentially pregnant adolescents and women with ionizing radiation. American College of Radiology Practice guidelines & technical standards. 2008
- Ratnapalan S, Bona N, Chandra K, Koren G. Physicians' perceptions of teratogenic risk associated with radiography and CT during early pregnancy. AJR Am J Roentgenol 2004; 182 (05) 1107-1109
- Doing WC, Do WY. Radiation Exposure and Pregnancy, Health Physics Society Fact Sheet https://hps.org/documents/pregnancy_fact_sheet.pdf
- Wang PI, Chong ST, Kielar AZ. et al. Imaging of pregnant and lactating patients: part 1, evidence-based review and recommendations. AJR Am J Roentgenol 2012; 198 (04) 778-784
- Valentin J. Chapter 3. Effects of in utero irradiation. Ann ICRP 2000; 30 (01) 9-12
- National Research Council. Health Risks from Exposure to Low Levels of Ionizing Radiation: BEIR VII Phase 2. Washington, DC: The National Academies Press;
- American College of Obstetricians and Gynecologists. Guidelines for diagnostic imaging during pregnancy. ACOG Comm Opin 1995; (Sep): 158
- Khaddour K, Hana CK, Mewawalla P. Hematopoietic Stem Cell Transplantation. Treasure Island, FL: StatPearls Publishing; InStatPearls [internet] 2021 Jul 25.
- Saad A, de Lima M, Anand S. et al. Hematopoietic cell transplantation, version 2.2020, NCCN clinical practice guidelines in oncology. J Natl Compr Canc Netw 2020; 18 (05) 599-634
- D'Souza A, Pasquini M, Spellecy R. Is 'informed consent' an 'understood consent' in hematopoietic cell transplantation?. Bone Marrow Transplant 2015; 50 (01) 10-14
- Pandey T, Maximin S, Bhargava P. Imaging of complications from hematopoietic stem cell transplant. Indian J Radiol Imaging 2014; 24 (04) 327-338
- Aljurf M, Snowden JA, Hayden P, Orchard KH, McGrath E. Quality Management and Accreditation in Hematopoietic Stem Cell Transplantation and Cellular Therapy: The JACIE Guide. Springer Nature; 2021
- Maxim LD, Niebo R, Utell MJ. Screening tests: a review with examples. Inhal Toxicol 2014; 26 (13) 811-828
- Kopans DB, Monsees B, Smith R, Feig S. Ten criteria for effective screening. AJR Am J Roentgenol 2002; 178 (02) 508-510
- Croswell JM, Ransohoff DF, Kramer BS. Principles of cancer screening: lessons from history and study design issues. Semin Oncol 2010; 37 (03) 202-215 WB Saunders
- Ferlay J, Shin HR, Bray F, Forman D, Mathers C, Parkin DM. Estimates of worldwide burden of cancer in 2008: GLOBOCAN 2008. Int J Cancer 2010; 127 (12) 2893-2917
- Bashar MA, Aggarwal AK. Organizing a cancer screening camp in low-resource settings: experience from North India. Indian J Med Paed Oncol 2019; 40 (S01): S194-S195
- Bray F, Ferlay J, Soerjomataram I, Siegel RL, Torre LA, Jemal A. Global cancer statistics 2018: GLOBOCAN estimates of incidence and mortality worldwide for 36 cancers in 185 countries. CA Cancer J Clin 2018; 68 (06) 394-424
- Takkar N, Kochhar S, Garg P, Pandey AK, Dalal UR, Handa U. Screening methods (clinical breast examination and mammography) to detect breast cancer in women aged 40-49 years. J Midlife Health 2017; 8 (01) 2-10
- Gradishar WJ, Anderson BO, Abraham J. et al. Breast cancer, version 3.2020, NCCN clinical practice guidelines in oncology. J Natl Compr Canc Netw 2020; 18 (04) 452-478
- Siu AL, Bibbins-Domingo K, Grossman DC, LeFevre ML. U.S. Preventive Services Task Force. Convergence and divergence around breast cancer screening. Ann Intern Med 2016; 164 (04) 301-302
- Qaseem A, Lin JS, Mustafa RA. et al; Clinical Guidelines Committee of the American College of Physicians. Screening for breast cancer in average-risk women: a guidance statement from the American College of Physicians. Ann Intern Med 2019; 170 (08) 547-560
- Marmot MG, Altman DG, Cameron DA, Dewar JA, Thompson SG, Wilcox M. The benefits and harms of breast cancer screening: an independent review. Br J Cancer 2013; 108 (11) 2205-2240
- Murthy NS, Chaudhry K, Nadayil D, Agarwal UK, Saxena S. Changing trends in incidence of breast cancer: Indian scenario. Indian J Cancer 2009; 46 (01) 73-74
- Keegan N, Goldgar C, Keahey D. Colorectal cancer and computed tomography colonography: a new screening option?. J Physician Assist Educ 2010; 21 (01) 35-42
- Singh S, Shrivastava JP, Dwivedi A. Breast cancer screening existence in India: A nonexisting reality. Indian J Med Paediatr Oncol 2015; 36 (04) 207-209
- Shulman LN, Willett W, Sievers A, Knaul FM. Breast cancer in developing countries: opportunities for improved survival. J Oncol 2010; 2010: 595167 DOI: 10.1155/2010/595167. . Epub 2010 Dec 29. PMID: 21253541; PMCID: PMC3021855
- Bleyer A, Welch HG. Effect of three decades of screening mammography on breast-cancer incidence. N Engl J Med 2012; 367 (21) 1998-2005
- Badwe RA, Gupta S. Mammographic screening for breast cancer: Are the chickens coming home to roost?. South Asian J Cancer 2013; 2 (01) 1-2
- Gupta A, Shridhar K, Dhillon PK. A review of breast cancer awareness among women in India: Cancer literate or awareness deficit?. Eur J Cancer 2015; 51 (14) 2058-2066
- Mittra I, Mishra GA, Singh S. et al. A cluster randomized, controlled trial of breast and cervix cancer screening in Mumbai, India: methodology and interim results after three rounds of screening. Int J Cancer 2010; 126 (04) 976-984
- Bluekens AM, Holland R, Karssemeijer N, Broeders MJ, den Heeten GJ. Comparison of digital screening mammography and screen-film mammography in the early detection of clinically relevant cancers: a multicenter study. Radiology 2012; 265 (03) 707-714
- Mercado CL. BI-RADS update. Radiol Clin North Am 2014; 52 (03) 481-487
- D'Orsi CJ. Imaging for the diagnosis and management of ductal carcinoma in situ. J Natl Cancer Inst Monogr 2010; 2010 (41) 214-217
- Uematsu T. The emerging role of breast tomosynthesis. Breast Cancer 2013; 20 (03) 204-212
- Berg WA, Blume JD, Cormack JB. et al; ACRIN 6666 Investigators. Combined screening with ultrasound and mammography vs mammography alone in women at elevated risk of breast cancer. JAMA 2008; 299 (18) 2151-2163
- Berg WA, Bandos AI, Mendelson EB, Lehrer D, Jong RA, Pisano ED. Ultrasound as the primary screening test for breast cancer: analysis from ACRIN 6666. J Natl Cancer Inst 2015; 108 (04) djv367
- Agrawal A, Tripathi P, Sahu A, Daftary J. Breast screening revisited. J Family Med Prim Care 2014; 3 (04) 340-344
- Saadatmand S, Geuzinge HA, Rutgers EJT. et al; FaMRIsc study group. MRI versus mammography for breast cancer screening in women with familial risk (FaMRIsc): a multicentre, randomised, controlled trial. Lancet Oncol 2019; 20 (08) 1136-1147
- Torre LA, Siegel RL, Jemal A. Lung cancer statistics. Adv Exp Med Biol 2016; 893: 1-19
- Behera D, Balamugesh T. Lung cancer in India. Indian J Chest Dis Allied Sci 2004; 46 (04) 269-281
- Moyer VA. U.S. Preventive Services Task Force. Screening for lung cancer: U.S. Preventive Services Task Force recommendation statement. Ann Intern Med 2014; 160 (05) 330-338
- Humphrey LL, Deffebach M, Pappas M. et al. Screening for lung cancer with low-dose computed tomography: a systematic review to update the US Preventive services task force recommendation. Ann Intern Med 2013; 159 (06) 411-420
- Wood DE, Kazerooni EA, Baum SL. et al. Lung cancer screening, version 3.2018, NCCN clinical practice guidelines in oncology. J Natl Compr Canc Netw 2018; 16 (04) 412-441
- Issa IA, Noureddine M. Colorectal cancer screening: an updated review of the available options. World J Gastroenterol 2017; 23 (28) 5086-5096
- Li D. Recent advances in colorectal cancer screening. Chronic Dis Transl Med 2018; 4 (03) 139-147
- Burt RW, Barthel JS, Dunn KB. et al; NCCN. NCCN clinical practice guidelines in oncology. Colorectal cancer screening. J Natl Compr Canc Netw 2010; 8 (01) 8-61
- Chan CK, Aimagambetova G, Ukybassova T, Kongrtay K, Azizan A. Human papillomavirus infection and cervical cancer: epidemiology, screening, and vaccination—review of current perspectives. J Oncol 2019; 2019: 3257939
- Walboomers JM, Jacobs MV, Manos MM. et al. Human papillomavirus is a necessary cause of invasive cervical cancer worldwide. J Pathol 1999; 189 (01) 12-19
- Saslow D, Solomon D, Lawson HW. et al; American Cancer Society, American Society for Colposcopy and Cervical Pathology, American Society for Clinical Pathology. American Cancer Society, American Society for Colposcopy and Cervical Pathology, and American Society for Clinical Pathology screening guidelines for the prevention and early detection of cervical cancer. Am J Clin Pathol 2012; 137 (04) 516-542
- Srivastava AN, Misra JS, Srivastava S, Das BC, Gupta S. Cervical cancer screening in rural India: status and current concepts. Indian J Med Res 2018; 148 (06) 687-696
- Rawla P. Epidemiology of prostate cancer. World J Oncol 2019; 10 (02) 63-89
- Mohler JL, Antonarakis ES, Armstrong AJ. et al. Prostate cancer, version 2.2019, NCCN clinical practice guidelines in oncology. J Natl Compr Canc Netw 2019; 17 (05) 479-505
- Daly MB, Pilarski R, Yurgelun MB. et al. NCCN guidelines insights: genetic/familial high-risk assessment: breast, ovarian, and pancreatic, version 1.2020. J Natl Compr Canc Netw 2020; 18 (04) 380-391
- Mahajan A, Vaidya T, Gupta A, Rane S, Gupta S. Artificial intelligence in healthcare in developing nations: the beginning of a transformative journey. Cancer Res, Stat Treat 2019; 2 (02) 182-189
- Bothra M, Mahajan A. Mining artificial intelligence in oncology: Tata Memorial Hospital journey. Cancer Res Stat Treat 2020; 3: 622-624
- Shur JD, Doran SJ, Kumar S. et al. Radiomics in oncology: a practical guide. Radiographics 2021; 41 (06) 1717-1732
- Tran KA, Kondrashova O, Bradley A, Williams ED, Pearson JV, Waddell N. Deep learning in cancer diagnosis, prognosis and treatment selection. Genome Med 2021; 13 (01) 152
- Montagnon E, Cerny M, Cadrin-Chênevert A. et al. Deep learning workflow in radiology: a primer. Insights Imaging 2020; 11 (01) 22
- Tseng H-H, Wei L, Cui S, Luo Y, Ten Haken RK, El Naqa I. Machine learning and imaging informatics in oncology. Oncology 2020; 98 (06) 344-362
- Choy G, Khalilzadeh O, Michalski M. et al. Current applications and future impact of machine learning in radiology. Radiology 2018; 288 (02) 318-328
- Wang S, Summers RM. Machine learning and radiology. Med Image Anal 2012; 16 (05) 933-951
- Cheng PM, Montagnon E, Yamashita R. et al. Deep learning: an update for radiologists. Radiographics 2021; 41 (05) 1427-1445
- Rieke N, Hancox J, Li W. et al. The future of digital health with federated learning. NPJ Digit Med 2020; 3 (01) 119
- Accessed March 26, 2022 at: https://www.javatpoint.com/machine-learning-vs-deep-learning
- Sapate S, Talbar S, Mahajan A, Sable N, Desai S, Thakur M. Breast cancer diagnosis using abnormalities on ipsilateral views of digital mammograms. Biocybern Biomed Eng 2020; 40 (01) 290-305
- Sapate SG, Mahajan A, Talbar SN, Sable N, Desai S, Thakur M. Radiomics based detection and characterization of suspicious lesions on full field digital mammograms. Comput Meth Prog Biomed 2018; 163: 1-20
- Baid U, Ghodasara S, Mohan S. et al. The RSNA-ASNR-MICCAI BraTS 2021 benchmark on brain tumor segmentation and radiogenomic classification. arXiv preprint arXiv:2107.02314. 2021 Jul 5.
- Baid U, Talbar S, Rane S. et al. A novel approach for fully automatic intra-tumor segmentation with 3D U-net architecture for gliomas. Front Comput Neurosci 2020; 14: 10
- Mehta R, Filos A, Baid U, Sako C, McKinley R, Rebsamen M, Dätwyler K, Meier R, Radojewski P, Krishnan Murugesan G, Nalawade S. QU-BraTS: MICCAI BraTS 2020 challenge on quantifying uncertainty in brain tumor segmentation–analysis of ranking metrics and benchmarking results. arXiv e-prints. 2021 Dec:arXiv-2112.
- Pati S, Baid U, Zenk M. et al. The federated tumor segmentation (FETS) challenge. arXiv preprint arXiv:2105.05874. 2021 May 12.
- Singadkar G, Mahajan A, Thakur M, Talbar S. Deep deconvolutional residual network based automatic lung nodule segmentation. J Digit Imaging 2020; 33 (03) 678-684
- Singadkar G, Mahajan A, Thakur M, Talbar S. Automatic lung segmentation for the inclusion of juxtapleural nodules and pulmonary vessels using curvature based border correction. J King Saud Univ-Comput Informat Sci 2021; 33 (08) 975-987
- Kumar YR, Muthukrishnan NM, Mahajan A. et al. Statistical parameter-based automatic liver tumor segmentation from abdominal CT scans: a potential radiomic signature. Procedia Comput Sci 2016; 93: 446-452
- Rela M, Krishnaveni BV, Kumar P, Lakshminarayana G. Computerized segmentation of liver tumor using integrated fuzzy level set method. AIP Conf Proc 2021; 2358 (01) 60001
- Hambarde P, Talbar SN, Sable N, Mahajan A, Chavan SS, Thakur M. Radiomics for peripheral zone and intra-prostatic urethra segmentation in MR imaging. Biomed Signal Process Control 2019; 51: 19-29
- Davatzikos C, Barnholtz-Sloan JS, Bakas S. et al. AI-based prognostic imaging biomarkers for precision neuro-oncology: the ReSPOND consortium. Neuro-oncol 2020; 22 (06) 886-888
- Bakas S, Reyes M, Jakab A. et al. Identifying the best machine learning algorithms for brain tumor segmentation, progression assessment, and overall survival prediction in the BRATS challenge. arXiv preprint arXiv:1811.02629. 2018 Nov 5.
- Baid U, Rane SU, Talbar S. et al. Overall survival prediction in glioblastoma with radiomic features using machine learning. Front Comput Neurosci 2020; 14: 61
- Akbari H, Mohan S, Garcia JA. et al. Prediction of glioblastoma cellular infiltration and recurrence using machine learning and multi-parametric MRI analysis: results from the multi-institutional respond consortium. Neuro-oncol 2021; 23 (Supplement_6): vi132-vi133
- Mantarro A, Scalise P, Neri E. Imaging biobanks, big data, and population-based imaging biomarkers. In: Imaging Biomarkers: Development and Clinical Integration. 2017: 153-157
- Tang A, Tam R, Cadrin-Chênevert A. et al; Canadian Association of Radiologists (CAR) Artificial Intelligence Working Group. Canadian Association of Radiologists white paper on artificial intelligence in radiology. Can Assoc Radiol J 2018; 69 (02) 120-135
- van Timmeren JE, Cester D, Tanadini-Lang S, Alkadhi H, Baessler B. Radiomics in medical imaging-“how-to” guide and critical reflection. Insights Imaging 2020; 11 (01) 91
- Rizzo S, Botta F, Raimondi S. et al. Radiomics: the facts and the challenges of image analysis. Eur Radiol Exp 2018; 2 (01) 36
- Vial A, Stirling D, Field M. et al. The role of deep learning and radiomic feature extraction in cancer-specific predictive modelling: a review. Translational Cancer Research 2018; 7 (03) 803-816
- Geis JR, Brady A, Wu CC. et al. Ethics of artificial intelligence in radiology: summary of the joint European and North American multisociety statement. Insights Imaging 2019; 10 (01) 101
- Accessed October 20, 2022 at: https://www.cancerimagingarchive.net/
- Accessed October 20, 2022 at: https://www.rcr.ac.uk/sites/default/files/abstract_posters/a_white_paper_on_artificial_intelligence_in_radiology_getting_over_the_hype.pdf
- Bossuyt PM, Reitsma JB, Bruns DE. et al; STARD Group. STARD 2015: an updated list of essential items for reporting diagnostic accuracy studies. Clin Chem 2015; 61 (12) 1446-1452
- Collins GS, Reitsma JB, Altman DG, Moons KG. Transparent reporting of a multivariable prediction model for individual prognosis or diagnosis (TRIPOD): the TRIPOD statement. Br J Surg 2015; 102 (03) 148-158
- Last accessed October 20, 2022 at: https://www.rcr.ac.uk/posts/rcr-position-statement-artificial-intelligence
- Lambin P, Leijenaar RTH, Deist TM. et al. Radiomics: the bridge between medical imaging and personalized medicine. Nat Rev Clin Oncol 2017; 14 (12) 749-762
- Accessed October 10, 2022 at: https://www.niti.gov.in/sites/default/files/2021-02/Responsible-AI-22022021.pdf
- Akinci D'Antonoli T. Ethical considerations for artificial intelligence: an overview of the current radiology landscape. Diagn Interv Radiol 2020; 26 (05) 504-511
- Kourou K, Exarchos KP, Papaloukas C, Sakaloglou P, Exarchos T, Fotiadis DI. Applied machine learning in cancer research: a systematic review for patient diagnosis, classification and prognosis. Comput Struct Biotechnol J 2021; 19: 5546-5555
- Kumar Y, Gupta S, Singla R, Hu Y-C. A systematic review of artificial intelligence techniques in cancer prediction and diagnosis. Arch Comput Methods Eng 2022; 29 (04) 2043-2070
- Accessed October
20, 2022 at: https://car.ca/news/new-car-white-paper-on-ai-provides-guidance-on-de-identification-of-medical-imaging-data/
Address for correspondence
Dr. Abhishek Mahajan, MDDepartment of Radiology, The Clatterbridge Cancer Centre NHS Foundation TrustLiverpool, L7 8YAUnited KingdomEmail: abhishek.mahajan@nhs.netPublication History
Article published online:
06 March 2023© 2023. The Author(s). This is an open access article published by Thieme under the terms of the Creative Commons Attribution License, permitting unrestricted use, distribution, and reproduction so long as the original work is properly cited. (https://creativecommons.org/licenses/by/4.0/)
Thieme Medical and Scientific Publishers Pvt. Ltd.
A-12, 2nd Floor, Sector 2, Noida-201301 UP, India
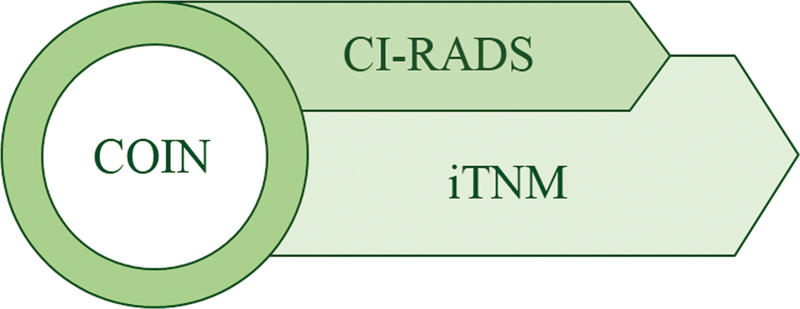
| Figure 1:
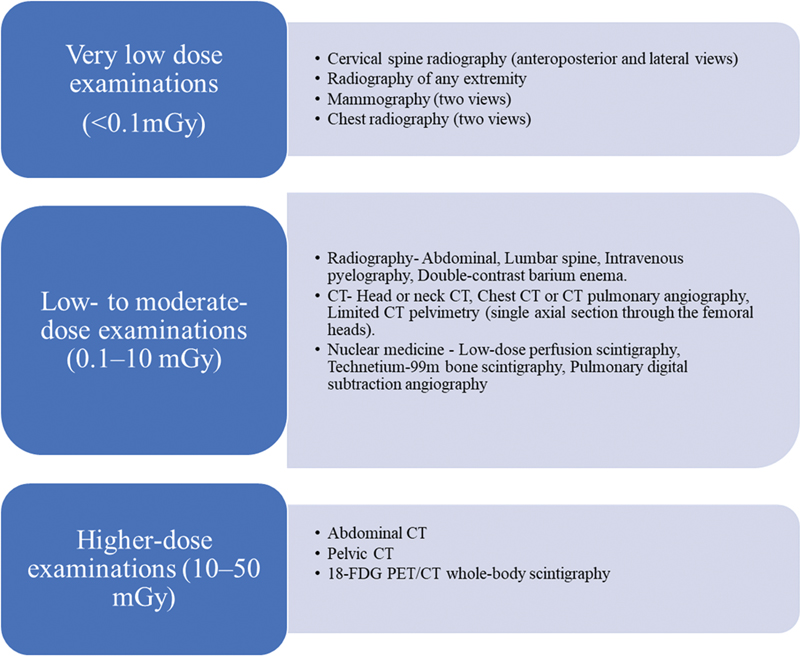
| Figure 1:Radiation doses associated with common radiologic examinations.
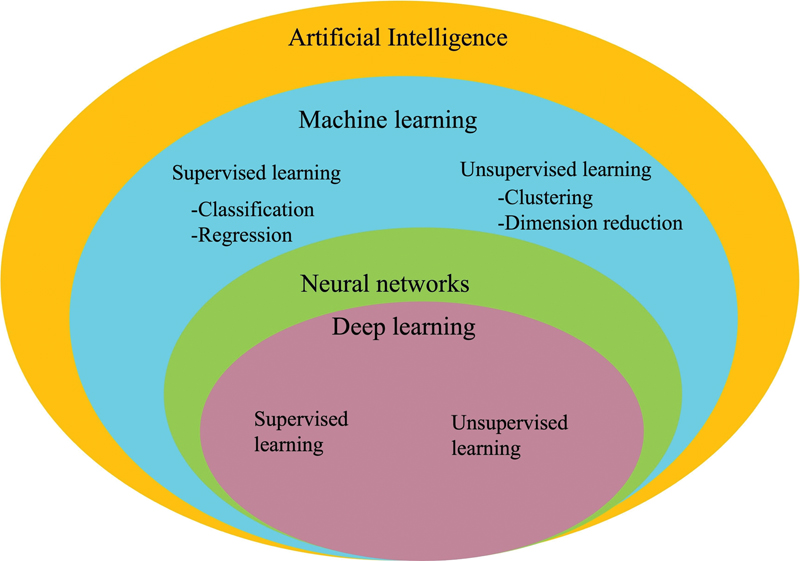
| Figure 2:Relationship between artificial intelligence, machine learning (ML), neural network, and deep learning (DL).

| Figure 3:Applications of artificial intelligence in oncological imaging.
References
- ung H, Ferlay J, Siegel RL. et al. Global Cancer Statistics 2020: GLOBOCAN estimates of incidence and mortality worldwide for 36 cancers in 185 countries. CA Cancer J Clin 2021; 71 (03) 209-249
- iles K. Can imaging help improve the survival of cancer patients?. Cancer Imaging 2011; 11 Spec No A (1A): S86-S92
- ard ZJ, Scott AM, Hricak H. et al. Estimating the impact of treatment and imaging modalities on 5-year net survival of 11 cancers in 200 countries: a simulation-based analysis. Lancet Oncol 2020; 21 (08) 1077-1088
- immermans S, Mauck A. The promises and pitfalls of evidence-based medicine. Health Aff (Millwood) 2005; 24 (01) 18-28
- deedo A, Van Schil P, Corthouts B, Van Mieghem F, Van Meerbeeck J, Van Marck E. Comparison of imaging TNM [(i)TNM] and pathological TNM [pTNM] in staging of bronchogenic carcinoma. Eur J Cardiothorac Surg 1997; 12 (02) 224-227
- ahajan A, Suthar M, Agarwal U, Shukla S, Thiagarajan S, Sable N. Imaging-based T stage (iT) as a predictive and prognostic marker for outcome in T4 stage tongue carcinomas: a narrative review. Cancer Res Stat Treat 2021; 4 (04) 677-683
- rommhold J, Jocham D, Doehn C. Wie gut ist die Korrelation zwischen klinischen und pathologischen TNM-Stadien bei Nierentumoren?. [How accurate is the correlation between clinical and pathological TNM stages in rena l tumours?] Aktuelle Urol 2011;42(4):247–251. German. doi: 10.1055/s-0031-1271392. Epub 2011 Jun 29
- n JY, Unsdorfer KML, Weinreb JC. BI-RADS, C-RADS, CAD-RADS, LI-RADS, Lung-RADS, NI-RADS, O-RADS, PI-RADS, TI-RADS: Reporting and Data Systems. Radiographics 2019; 39 (05) 1435-1436
- ccessed December 30, 2022 at: https://www.acr.org/Clinical-Resources/Reporting-and-Data-Systems/Bi-Rads
- Mahajan A. Synoptic reporting in lung cancers using Lung Cancer Reporting and Data System (LC-RADS): the road ahead for standardization of imaging in lung cancer staging. Cancer Res Stat Treat 2021; 4 (01) 61-66
- Mahajan A, Agarwal U, Padashetty S. et al. A narrative review of the role of cross-sectional imaging in the management of thyroid carcinoma: imaging guidelines and T-CIRADS. Cancer Research, Statistics, and Treatment 2022; 5 (03) 490-498
- Mahajan A, Agarwal U, Gupta A. et al. Synoptic reporting in head and neck cancers—Head and Neck Cancer Imaging Reporting and Data Systems (HN-CIRADS): the journey ahead for standardization of imaging in head and neck cancer staging. Cancer Res Stat Treat 2022; 5 (02) 322-330
- Ayer T, Chen Q, Burnside ES. Artificial neural networks in mammography interpretation and diagnostic decision making. Comput Math Methods Med 2013; 2013: 832509
- Steyerberg EW. Clinical Prediction Models: A Practical Approach to Development, Validation, and Updating. New York: Springer; 2009
- Carrino JA, Ohno-Machado L. Development of radiology prediction models using feature analysis. Acad Radiol 2005; 12 (04) 415-421
- European Society of Radiology (ESR). ESR position paper on imaging biobanks. Insights Imaging 2015; 6 (04) 403-410
- Vaidya T, Agrawal A, Mahajan S, Thakur MH, Mahajan A. The continuing evolution of molecular functional imaging in clinical oncology: the road to precision medicine and radiogenomics (Part II). Mol Diagn Ther 2019; 23 (01) 27-51 Erratum in: Mol Diagn Ther. 2018 Nov 20; PMID: 30387041
- Miller AB, Hoogstraten B, Staquet M, Winkler A. Reporting results of cancer treatment. Cancer 1981; 47 (01) 207-214
- Therasse P, Arbuck SG, Eisenhauer EA. et al. New guidelines to evaluate the response to treatment in solid tumors. European Organization for Research and Treatment of Cancer, National Cancer Institute of the United States, National Cancer Institute of Canada. J Natl Cancer Inst 2000; 92 (03) 205-216
- Kim JH. Comparison of the EORTC criteria and PERCIST in solid tumors: a pooled analysis and review. Oncotarget 2016; 7 (36) 58105-58110
- Mahajan A, Cook G. Clinical applications of PET/CT in oncology. In: Basic Science of PET Imaging; 2017: 429
- Mahajan A, Cook G. Physiologic and molecular basis of PET in cancer imaging. In: Basic Science of PET Imaging; 2017: 399-427
- Ko CC, Yeh LR, Kuo YT, Chen JH. Imaging biomarkers for evaluating tumor response: RECIST and beyond. Biomark Res 2021; 9 (01) 52
- Somarouthu B, Lee SI, Urban T, Sadow CA, Harris GJ, Kambadakone A. Immune-related tumour response assessment criteria: a comprehensive review. Br J Radiol 2018; 91 (1084): 20170457
- Choi H, Charnsangavej C, Faria SC. et al. Correlation of computed tomography and positron emission tomography in patients with metastatic gastrointestinal stromal tumor treated at a single institution with imatinib mesylate: proposal of new computed tomography response criteria. J Clin Oncol 2007; 25 (13) 1753-1759
- Lencioni R, Llovet JM. Modified RECIST (mRECIST) assessment for hepatocellular carcinoma. Semin Liver Dis 2010; 30 (01) 52-60
- Wahl RL, Jacene H, Kasamon Y, Lodge MA. From RECIST to PERCIST: evolving considerations for PET response criteria in solid tumors. J Nucl Med 2009; 50 (Suppl. 01) 122S-150S
- Chinot OL, Macdonald DR, Abrey LE, Zahlmann G, Kerloëguen Y, Cloughesy TF. Response assessment criteria for glioblastoma: practical adaptation and implementation in clinical trials of antiangiogenic therapy. Curr Neurol Neurosci Rep 2013; 13 (05) 347
- Cheson BD, Pfistner B, Juweid ME. et al; International Harmonization Project on Lymphoma. Revised response criteria for malignant lymphoma. J Clin Oncol 2007; 25 (05) 579-586
- Abdel-Sattar MH, Abdelaziz O, Othman AO, El-Refaei SM. The use of Deauville criteria in follow-up assessment of response to therapy in extra-nodal Non-Hodgkin's lymphoma. Egypt J Radiol Nucl Med 2018; 49 (01) 209-215
- Nielsen N, Nielsen A, Gerke O, Juul-Jensen K, Larsen T, Hildebrandt M. A head-to-head comparison of the Lugano Classification and PERCIST for FDG-PET/CT response assessment in diffuse large B-cell lymphoma. J Nucl Med 2021; 62 (Suppl 1): 1688
- Costelloe CM, Chuang HH, Madewell JE, Ueno NT. Cancer response criteria and bone metastases: RECIST 1.1, MDA and PERCIST. J Cancer 2010; 1: 80-92
- Aykan NF, Özatlı T. Objective response rate assessment in oncology: current situation and future expectations. World J Clin Oncol 2020; 11 (02) 53-73
- Tirkes T, Hollar MA, Tann M, Kohli MD, Akisik F, Sandrasegaran K. Response criteria in oncologic imaging: review of traditional and new criteria. Radiographics 2013; 33 (05) 1323-1341
- Ranganathan P, Sengar M, Chinnaswamy G. et al; National Cancer Grid of India. Impact of COVID-19 on cancer care in India: a cohort study. Lancet Oncol 2021; 22 (07) 970-976
- Richards M, Anderson M, Carter P, Ebert BL, Mossialos E. The impact of the COVID-19 pandemic on cancer care. Nat Can 2020; 1 (06) 565-567
- Johannesen TB, Smeland S, Aaserud S. et al. COVID-19 in cancer patients, risk factors for disease and adverse outcome, a population-based study from Norway. Front Oncol 2021; 11: 652535
- Haghighat S, Dehghani M. COVID-19 in immunosuppressed and cancer patients: a review. Asian Pacific J Cancer Care 2020; 5 (S1): 69-73
- Fillmore NR, La J, Szalat RE. et al. Prevalence and outcome of COVID-19 infection in cancer patients: A National Veterans Affairs Study. J Natl Cancer Inst 2021; 113 (06) 691-698
- Aljondi R, Alghamdi S. Diagnostic value of imaging modalities for COVID-19: scoping review. J Med Internet Res 2020; 22 (08) e19673
- Cleverley J, Piper J, Jones MM. The role of chest radiography in confirming covid-19 pneumonia. BMJ 2020; 370: m2426
- Nnaji CA, Moodley J. Impact of the COVID-19 pandemic on cancer diagnosis, treatment and research in African health systems: a review of current evidence and contextual perspectives. Ecancermedicalscience 2021; 15: 1170
- Kulkarni T, Sharma P, Pande P, Agrawal R, Rane S, Mahajan A. COVID-19: a review of protective measures. Cancer Res, Statistics Treat 2020; 3 (02) 244-253
- Curigliano G, Banerjee S, Cervantes A. et al; Panel Members. Managing cancer patients during the COVID-19 pandemic: an ESMO multidisciplinary expert consensus. Ann Oncol 2020; 31 (10) 1320-1335
- Boettcher AN, Hammoud DA, Weinberg JB, Agarwal P, Mendiratta-Lala M, Luker GD. Cancer imaging and patient care during the COVID-19 pandemic. Radiol Imaging Cancer 2020; 2 (06) e200058
- McCollough CH, Schueler BA, Atwell TD. et al. Radiation exposure and pregnancy: when should we be concerned?. Radiographics 2007; 27 (04) 909-917 , discussion 917–918
- Patel SJ, Reede DL, Katz DS, Subramaniam R, Amorosa JK. Imaging the pregnant patient for nonobstetric conditions: algorithms and radiation dose considerations. Radiographics 2007; 27 (06) 1705-1722
- American Institute of Ultrasound in Medicine. Statement on the safe use of Doppler ultrasound during 11–14 week scans (or earlier in pregnancy). Laurel, MD: AIUM; 2011. Accessed December 30, 2022 at: http://www.aium.org/officialStatements/42
- Chen MM, Coakley FV, Kaimal A, Laros Jr RK. Guidelines for computed tomography and magnetic resonance imaging use during pregnancy and lactation. Obstet Gynecol 2008; 112 (2 Pt 1): 333-340
- Leyendecker JR, Gorengaut V, Brown JJ. MR imaging of maternal diseases of the abdomen and pelvis during pregnancy and the immediate postpartum period. Radiographics 2004; 24 (05) 1301-1316
- Kanal E, Barkovich AJ, Bell C. et al; Expert Panel on MR Safety. ACR guidance document on MR safe practices: 2013. J Magn Reson Imaging 2013; 37 (03) 501-530
- De Santis M, Straface G, Cavaliere AF, Carducci B, Caruso A. Gadolinium periconceptional exposure: pregnancy and neonatal outcome. Acta Obstet Gynecol Scand 2007; 86 (01) 99-101
- Ray JG, Vermeulen MJ, Bharatha A, Montanera WJ, Park AL. Association between MRI exposure during pregnancy and fetal and childhood outcomes. JAMA 2016; 316 (09) 952-961
- Sachs HC. Committee On Drugs. The transfer of drugs and therapeutics into human breast milk: an update on selected topics. Pediatrics 2013; 132 (03) e796-e809
- De Santis M, Cesari E, Nobili E, Straface G, Cavaliere AF, Caruso A. Radiation effects on development. Birth Defects Res C Embryo Today 2007; 81 (03) 177-182
- Brent RL. Saving lives and changing family histories: appropriate counseling of pregnant women and men and women of reproductive age, concerning the risk of diagnostic radiation exposures during and before pregnancy. Am J Obstet Gynecol 2009; 200 (01) 4-24
- Wagner LK, Applegate K. American College of Radiology. ACR practice guideline for imaging pregnant or potentially pregnant adolescents and women with ionizing radiation. American College of Radiology Practice guidelines & technical standards. 2008
- Ratnapalan S, Bona N, Chandra K, Koren G. Physicians' perceptions of teratogenic risk associated with radiography and CT during early pregnancy. AJR Am J Roentgenol 2004; 182 (05) 1107-1109
- Doing WC, Do WY. Radiation Exposure and Pregnancy, Health Physics Society Fact Sheet https://hps.org/documents/pregnancy_fact_sheet.pdf
- Wang PI, Chong ST, Kielar AZ. et al. Imaging of pregnant and lactating patients: part 1, evidence-based review and recommendations. AJR Am J Roentgenol 2012; 198 (04) 778-784
- Valentin J. Chapter 3. Effects of in utero irradiation. Ann ICRP 2000; 30 (01) 9-12
- National Research Council. Health Risks from Exposure to Low Levels of Ionizing Radiation: BEIR VII Phase 2. Washington, DC: The National Academies Press;
- American College of Obstetricians and Gynecologists. Guidelines for diagnostic imaging during pregnancy. ACOG Comm Opin 1995; (Sep): 158
- Khaddour K, Hana CK, Mewawalla P. Hematopoietic Stem Cell Transplantation. Treasure Island, FL: StatPearls Publishing; InStatPearls [internet] 2021 Jul 25.
- Saad A, de Lima M, Anand S. et al. Hematopoietic cell transplantation, version 2.2020, NCCN clinical practice guidelines in oncology. J Natl Compr Canc Netw 2020; 18 (05) 599-634
- D'Souza A, Pasquini M, Spellecy R. Is 'informed consent' an 'understood consent' in hematopoietic cell transplantation?. Bone Marrow Transplant 2015; 50 (01) 10-14
- Pandey T, Maximin S, Bhargava P. Imaging of complications from hematopoietic stem cell transplant. Indian J Radiol Imaging 2014; 24 (04) 327-338
- Aljurf M, Snowden JA, Hayden P, Orchard KH, McGrath E. Quality Management and Accreditation in Hematopoietic Stem Cell Transplantation and Cellular Therapy: The JACIE Guide. Springer Nature; 2021
- Maxim LD, Niebo R, Utell MJ. Screening tests: a review with examples. Inhal Toxicol 2014; 26 (13) 811-828
- Kopans DB, Monsees B, Smith R, Feig S. Ten criteria for effective screening. AJR Am J Roentgenol 2002; 178 (02) 508-510
- Croswell JM, Ransohoff DF, Kramer BS. Principles of cancer screening: lessons from history and study design issues. Semin Oncol 2010; 37 (03) 202-215 WB Saunders
- Ferlay J, Shin HR, Bray F, Forman D, Mathers C, Parkin DM. Estimates of worldwide burden of cancer in 2008: GLOBOCAN 2008. Int J Cancer 2010; 127 (12) 2893-2917
- Bashar MA, Aggarwal AK. Organizing a cancer screening camp in low-resource settings: experience from North India. Indian J Med Paed Oncol 2019; 40 (S01): S194-S195
- Bray F, Ferlay J, Soerjomataram I, Siegel RL, Torre LA, Jemal A. Global cancer statistics 2018: GLOBOCAN estimates of incidence and mortality worldwide for 36 cancers in 185 countries. CA Cancer J Clin 2018; 68 (06) 394-424
- Takkar N, Kochhar S, Garg P, Pandey AK, Dalal UR, Handa U. Screening methods (clinical breast examination and mammography) to detect breast cancer in women aged 40-49 years. J Midlife Health 2017; 8 (01) 2-10
- Gradishar WJ, Anderson BO, Abraham J. et al. Breast cancer, version 3.2020, NCCN clinical practice guidelines in oncology. J Natl Compr Canc Netw 2020; 18 (04) 452-478
- Siu AL, Bibbins-Domingo K, Grossman DC, LeFevre ML. U.S. Preventive Services Task Force. Convergence and divergence around breast cancer screening. Ann Intern Med 2016; 164 (04) 301-302
- Qaseem A, Lin JS, Mustafa RA. et al; Clinical Guidelines Committee of the American College of Physicians. Screening for breast cancer in average-risk women: a guidance statement from the American College of Physicians. Ann Intern Med 2019; 170 (08) 547-560
- Marmot MG, Altman DG, Cameron DA, Dewar JA, Thompson SG, Wilcox M. The benefits and harms of breast cancer screening: an independent review. Br J Cancer 2013; 108 (11) 2205-2240
- Murthy NS, Chaudhry K, Nadayil D, Agarwal UK, Saxena S. Changing trends in incidence of breast cancer: Indian scenario. Indian J Cancer 2009; 46 (01) 73-74
- Keegan N, Goldgar C, Keahey D. Colorectal cancer and computed tomography colonography: a new screening option?. J Physician Assist Educ 2010; 21 (01) 35-42
- Singh S, Shrivastava JP, Dwivedi A. Breast cancer screening existence in India: A nonexisting reality. Indian J Med Paediatr Oncol 2015; 36 (04) 207-209
- Shulman LN, Willett W, Sievers A, Knaul FM. Breast cancer in developing countries: opportunities for improved survival. J Oncol 2010; 2010: 595167 DOI: 10.1155/2010/595167. . Epub 2010 Dec 29. PMID: 21253541; PMCID: PMC3021855
- Bleyer A, Welch HG. Effect of three decades of screening mammography on breast-cancer incidence. N Engl J Med 2012; 367 (21) 1998-2005
- Badwe RA, Gupta S. Mammographic screening for breast cancer: Are the chickens coming home to roost?. South Asian J Cancer 2013; 2 (01) 1-2
- Gupta A, Shridhar K, Dhillon PK. A review of breast cancer awareness among women in India: Cancer literate or awareness deficit?. Eur J Cancer 2015; 51 (14) 2058-2066
- Mittra I, Mishra GA, Singh S. et al. A cluster randomized, controlled trial of breast and cervix cancer screening in Mumbai, India: methodology and interim results after three rounds of screening. Int J Cancer 2010; 126 (04) 976-984
- Bluekens AM, Holland R, Karssemeijer N, Broeders MJ, den Heeten GJ. Comparison of digital screening mammography and screen-film mammography in the early detection of clinically relevant cancers: a multicenter study. Radiology 2012; 265 (03) 707-714
- Mercado CL. BI-RADS update. Radiol Clin North Am 2014; 52 (03) 481-487
- D'Orsi CJ. Imaging for the diagnosis and management of ductal carcinoma in situ. J Natl Cancer Inst Monogr 2010; 2010 (41) 214-217
- Uematsu T. The emerging role of breast tomosynthesis. Breast Cancer 2013; 20 (03) 204-212
- Berg WA, Blume JD, Cormack JB. et al; ACRIN 6666 Investigators. Combined screening with ultrasound and mammography vs mammography alone in women at elevated risk of breast cancer. JAMA 2008; 299 (18) 2151-2163
- Berg WA, Bandos AI, Mendelson EB, Lehrer D, Jong RA, Pisano ED. Ultrasound as the primary screening test for breast cancer: analysis from ACRIN 6666. J Natl Cancer Inst 2015; 108 (04) djv367
- Agrawal A, Tripathi P, Sahu A, Daftary J. Breast screening revisited. J Family Med Prim Care 2014; 3 (04) 340-344
- Saadatmand S, Geuzinge HA, Rutgers EJT. et al; FaMRIsc study group. MRI versus mammography for breast cancer screening in women with familial risk (FaMRIsc): a multicentre, randomised, controlled trial. Lancet Oncol 2019; 20 (08) 1136-1147
- Torre LA, Siegel RL, Jemal A. Lung cancer statistics. Adv Exp Med Biol 2016; 893: 1-19
- Behera D, Balamugesh T. Lung cancer in India. Indian J Chest Dis Allied Sci 2004; 46 (04) 269-281
- Moyer VA. U.S. Preventive Services Task Force. Screening for lung cancer: U.S. Preventive Services Task Force recommendation statement. Ann Intern Med 2014; 160 (05) 330-338
- Humphrey LL, Deffebach M, Pappas M. et al. Screening for lung cancer with low-dose computed tomography: a systematic review to update the US Preventive services task force recommendation. Ann Intern Med 2013; 159 (06) 411-420
- Wood DE, Kazerooni EA, Baum SL. et al. Lung cancer screening, version 3.2018, NCCN clinical practice guidelines in oncology. J Natl Compr Canc Netw 2018; 16 (04) 412-441
- Issa IA, Noureddine M. Colorectal cancer screening: an updated review of the available options. World J Gastroenterol 2017; 23 (28) 5086-5096
- Li D. Recent advances in colorectal cancer screening. Chronic Dis Transl Med 2018; 4 (03) 139-147
- Burt RW, Barthel JS, Dunn KB. et al; NCCN. NCCN clinical practice guidelines in oncology. Colorectal cancer screening. J Natl Compr Canc Netw 2010; 8 (01) 8-61
- Chan CK, Aimagambetova G, Ukybassova T, Kongrtay K, Azizan A. Human papillomavirus infection and cervical cancer: epidemiology, screening, and vaccination—review of current perspectives. J Oncol 2019; 2019: 3257939
- Walboomers JM, Jacobs MV, Manos MM. et al. Human papillomavirus is a necessary cause of invasive cervical cancer worldwide. J Pathol 1999; 189 (01) 12-19
- Saslow D, Solomon D, Lawson HW. et al; American Cancer Society, American Society for Colposcopy and Cervical Pathology, American Society for Clinical Pathology. American Cancer Society, American Society for Colposcopy and Cervical Pathology, and American Society for Clinical Pathology screening guidelines for the prevention and early detection of cervical cancer. Am J Clin Pathol 2012; 137 (04) 516-542
- Srivastava AN, Misra JS, Srivastava S, Das BC, Gupta S. Cervical cancer screening in rural India: status and current concepts. Indian J Med Res 2018; 148 (06) 687-696
- Rawla P. Epidemiology of prostate cancer. World J Oncol 2019; 10 (02) 63-89
- Mohler JL, Antonarakis ES, Armstrong AJ. et al. Prostate cancer, version 2.2019, NCCN clinical practice guidelines in oncology. J Natl Compr Canc Netw 2019; 17 (05) 479-505
- Daly MB, Pilarski R, Yurgelun MB. et al. NCCN guidelines insights: genetic/familial high-risk assessment: breast, ovarian, and pancreatic, version 1.2020. J Natl Compr Canc Netw 2020; 18 (04) 380-391
- Mahajan A, Vaidya T, Gupta A, Rane S, Gupta S. Artificial intelligence in healthcare in developing nations: the beginning of a transformative journey. Cancer Res, Stat Treat 2019; 2 (02) 182-189
- Bothra M, Mahajan A. Mining artificial intelligence in oncology: Tata Memorial Hospital journey. Cancer Res Stat Treat 2020; 3: 622-624
- Shur JD, Doran SJ, Kumar S. et al. Radiomics in oncology: a practical guide. Radiographics 2021; 41 (06) 1717-1732
- Tran KA, Kondrashova O, Bradley A, Williams ED, Pearson JV, Waddell N. Deep learning in cancer diagnosis, prognosis and treatment selection. Genome Med 2021; 13 (01) 152
- Montagnon E, Cerny M, Cadrin-Chênevert A. et al. Deep learning workflow in radiology: a primer. Insights Imaging 2020; 11 (01) 22
- Tseng H-H, Wei L, Cui S, Luo Y, Ten Haken RK, El Naqa I. Machine learning and imaging informatics in oncology. Oncology 2020; 98 (06) 344-362
- Choy G, Khalilzadeh O, Michalski M. et al. Current applications and future impact of machine learning in radiology. Radiology 2018; 288 (02) 318-328
- Wang S, Summers RM. Machine learning and radiology. Med Image Anal 2012; 16 (05) 933-951
- Cheng PM, Montagnon E, Yamashita R. et al. Deep learning: an update for radiologists. Radiographics 2021; 41 (05) 1427-1445
- Rieke N, Hancox J, Li W. et al. The future of digital health with federated learning. NPJ Digit Med 2020; 3 (01) 119
- Accessed March 26, 2022 at: https://www.javatpoint.com/machine-learning-vs-deep-learning
- Sapate S, Talbar S, Mahajan A, Sable N, Desai S, Thakur M. Breast cancer diagnosis using abnormalities on ipsilateral views of digital mammograms. Biocybern Biomed Eng 2020; 40 (01) 290-305
- Sapate SG, Mahajan A, Talbar SN, Sable N, Desai S, Thakur M. Radiomics based detection and characterization of suspicious lesions on full field digital mammograms. Comput Meth Prog Biomed 2018; 163: 1-20
- Baid U, Ghodasara S, Mohan S. et al. The RSNA-ASNR-MICCAI BraTS 2021 benchmark on brain tumor segmentation and radiogenomic classification. arXiv preprint arXiv:2107.02314. 2021 Jul 5.
- Baid U, Talbar S, Rane S. et al. A novel approach for fully automatic intra-tumor segmentation with 3D U-net architecture for gliomas. Front Comput Neurosci 2020; 14: 10
- Mehta R, Filos A, Baid U, Sako C, McKinley R, Rebsamen M, Dätwyler K, Meier R, Radojewski P, Krishnan Murugesan G, Nalawade S. QU-BraTS: MICCAI BraTS 2020 challenge on quantifying uncertainty in brain tumor segmentation–analysis of ranking metrics and benchmarking results. arXiv e-prints. 2021 Dec:arXiv-2112.
- Pati S, Baid U, Zenk M. et al. The federated tumor segmentation (FETS) challenge. arXiv preprint arXiv:2105.05874. 2021 May 12.
- Singadkar G, Mahajan A, Thakur M, Talbar S. Deep deconvolutional residual network based automatic lung nodule segmentation. J Digit Imaging 2020; 33 (03) 678-684
- Singadkar G, Mahajan A, Thakur M, Talbar S. Automatic lung segmentation for the inclusion of juxtapleural nodules and pulmonary vessels using curvature based border correction. J King Saud Univ-Comput Informat Sci 2021; 33 (08) 975-987
- Kumar YR, Muthukrishnan NM, Mahajan A. et al. Statistical parameter-based automatic liver tumor segmentation from abdominal CT scans: a potential radiomic signature. Procedia Comput Sci 2016; 93: 446-452
- Rela M, Krishnaveni BV, Kumar P, Lakshminarayana G. Computerized segmentation of liver tumor using integrated fuzzy level set method. AIP Conf Proc 2021; 2358 (01) 60001
- Hambarde P, Talbar SN, Sable N, Mahajan A, Chavan SS, Thakur M. Radiomics for peripheral zone and intra-prostatic urethra segmentation in MR imaging. Biomed Signal Process Control 2019; 51: 19-29
- Davatzikos C, Barnholtz-Sloan JS, Bakas S. et al. AI-based prognostic imaging biomarkers for precision neuro-oncology: the ReSPOND consortium. Neuro-oncol 2020; 22 (06) 886-888
- Bakas S, Reyes M, Jakab A. et al. Identifying the best machine learning algorithms for brain tumor segmentation, progression assessment, and overall survival prediction in the BRATS challenge. arXiv preprint arXiv:1811.02629. 2018 Nov 5.
- Baid U, Rane SU, Talbar S. et al. Overall survival prediction in glioblastoma with radiomic features using machine learning. Front Comput Neurosci 2020; 14: 61
- Akbari H, Mohan S, Garcia JA. et al. Prediction of glioblastoma cellular infiltration and recurrence using machine learning and multi-parametric MRI analysis: results from the multi-institutional respond consortium. Neuro-oncol 2021; 23 (Supplement_6): vi132-vi133
- Mantarro A, Scalise P, Neri E. Imaging biobanks, big data, and population-based imaging biomarkers. In: Imaging Biomarkers: Development and Clinical Integration. 2017: 153-157
- Tang A, Tam R, Cadrin-Chênevert A. et al; Canadian Association of Radiologists (CAR) Artificial Intelligence Working Group. Canadian Association of Radiologists white paper on artificial intelligence in radiology. Can Assoc Radiol J 2018; 69 (02) 120-135
- van Timmeren JE, Cester D, Tanadini-Lang S, Alkadhi H, Baessler B. Radiomics in medical imaging-“how-to” guide and critical reflection. Insights Imaging 2020; 11 (01) 91
- Rizzo S, Botta F, Raimondi S. et al. Radiomics: the facts and the challenges of image analysis. Eur Radiol Exp 2018; 2 (01) 36
- Vial A, Stirling D, Field M. et al. The role of deep learning and radiomic feature extraction in cancer-specific predictive modelling: a review. Translational Cancer Research 2018; 7 (03) 803-816
- Geis JR, Brady A, Wu CC. et al. Ethics of artificial intelligence in radiology: summary of the joint European and North American multisociety statement. Insights Imaging 2019; 10 (01) 101
- Accessed October 20, 2022 at: https://www.cancerimagingarchive.net/
- Accessed October 20, 2022 at: https://www.rcr.ac.uk/sites/default/files/abstract_posters/a_white_paper_on_artificial_intelligence_in_radiology_getting_over_the_hype.pdf
- Bossuyt PM, Reitsma JB, Bruns DE. et al; STARD Group. STARD 2015: an updated list of essential items for reporting diagnostic accuracy studies. Clin Chem 2015; 61 (12) 1446-1452
- Collins GS, Reitsma JB, Altman DG, Moons KG. Transparent reporting of a multivariable prediction model for individual prognosis or diagnosis (TRIPOD): the TRIPOD statement. Br J Surg 2015; 102 (03) 148-158
- Last accessed October 20, 2022 at: https://www.rcr.ac.uk/posts/rcr-position-statement-artificial-intelligence
- Lambin P, Leijenaar RTH, Deist TM. et al. Radiomics: the bridge between medical imaging and personalized medicine. Nat Rev Clin Oncol 2017; 14 (12) 749-762
- Accessed October 10, 2022 at: https://www.niti.gov.in/sites/default/files/2021-02/Responsible-AI-22022021.pdf
- Akinci D'Antonoli T. Ethical considerations for artificial intelligence: an overview of the current radiology landscape. Diagn Interv Radiol 2020; 26 (05) 504-511
- Kourou K, Exarchos KP, Papaloukas C, Sakaloglou P, Exarchos T, Fotiadis DI. Applied machine learning in cancer research: a systematic review for patient diagnosis, classification and prognosis. Comput Struct Biotechnol J 2021; 19: 5546-5555
- Kumar Y, Gupta S, Singla R, Hu Y-C. A systematic review of artificial intelligence techniques in cancer prediction and diagnosis. Arch Comput Methods Eng 2022; 29 (04) 2043-2070
- Accessed October 20, 2022 at: https://car.ca/news/new-car-white-paper-on-ai-provides-guidance-on-de-identification-of-medical-imaging-data/